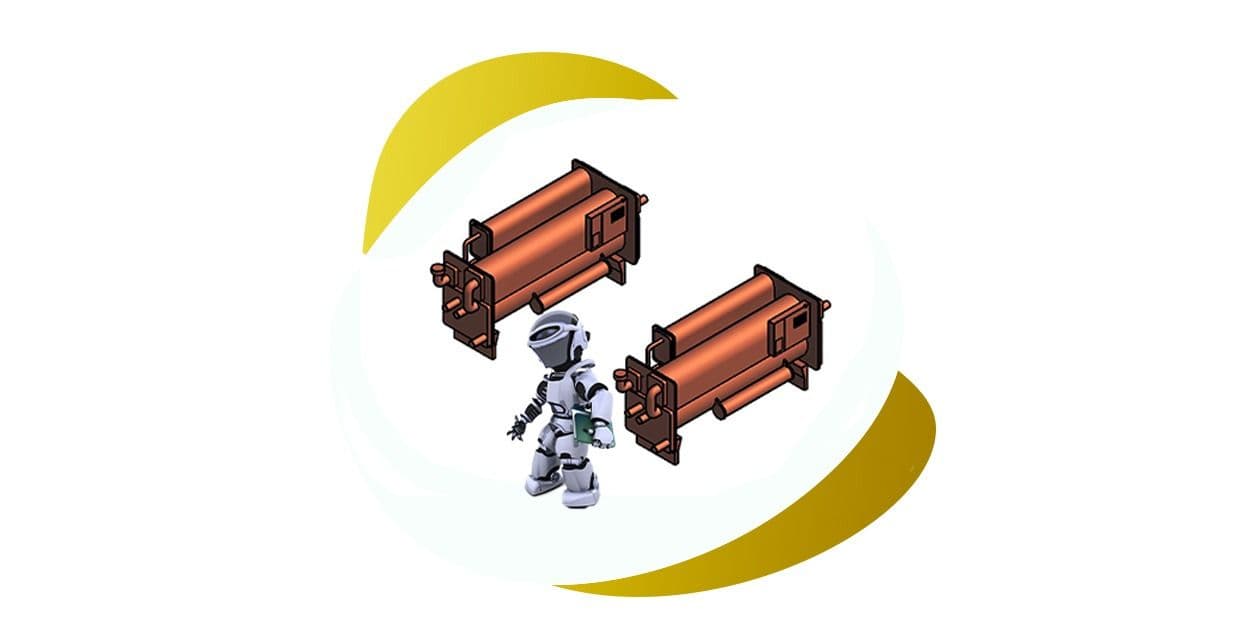
Global Optimization of Absorption Chiller Systems Using Genetic Algorithms and Neural Networks
Introduction
Currently, HVAC and refrigeration technologies in use globally consume approximately 20% of the electricity available. As industries strive to be more sustainable and efficient, they are actively seeking innovative solutions to improve their operations. Imagine if optimization models built with AI could potentially minimize the energy consumption of absorption chillers by 40%?
Absorption chiller systems are popular with regards to industrial cooling as well as HVAC applications because of their capability to make use of waste heat. Nonetheless, the systems experience issues like nonlinear thermodynamic behavior, energy inefficient operation, load demand variability, and cyclical energetic patterns. Furthermore, traditional optimization approaches do not seem to manage the above complexities in an effective manner.
This is the gap where Genetic Algorithms (GA) and Neural Networks (NN) step in to make a difference. GA, which is developed from ideas of natural selection, tries all possible solutions to determine the best operational parameters and optimum sequenced solutions. While NN focuses on continually improving these parameters through learning and real-time prediction. A combination of both approaches enable industries to create cooling systems that need minimal human intervention through self-optimizing capabilities.
Need for Improving Absorption Chiller Systems
The chillers are used to supply cooling to industrial plants and in HVAC systems. Unlike other forms of chillers, absorption chillers are based on thermodynamic cycles, which tend to be complex. These systems may face efficiency problems due to malfunctions that come with varying load conditions, changes in the energy demand, and other environmental factors. Global optimization guarantees that the absorption chillers function on peak value efficiency while cutting down on carbon footprint, energy, and maintenance costs.
Energy Use and its Effect on the Environment
Cooling systems are one of the largest users of energy in the world. HVAC and refrigerator systems use approximately 20% of global electricity according to research done by the International Energy Agency (IEA). Businesses and industrial plants can optimize absorption chillers to reduce energy consumption by 30-40%, savings which would amount to millions of dollars each year.
Optimization of chillers has other advantages like reducing the emission of greenhouse gases. Global warming is an area of great concern and traditional cooling systems that are used are powered by fossil fuels, the optimization through AI makes it easier for industries to work towards sustainable goals.
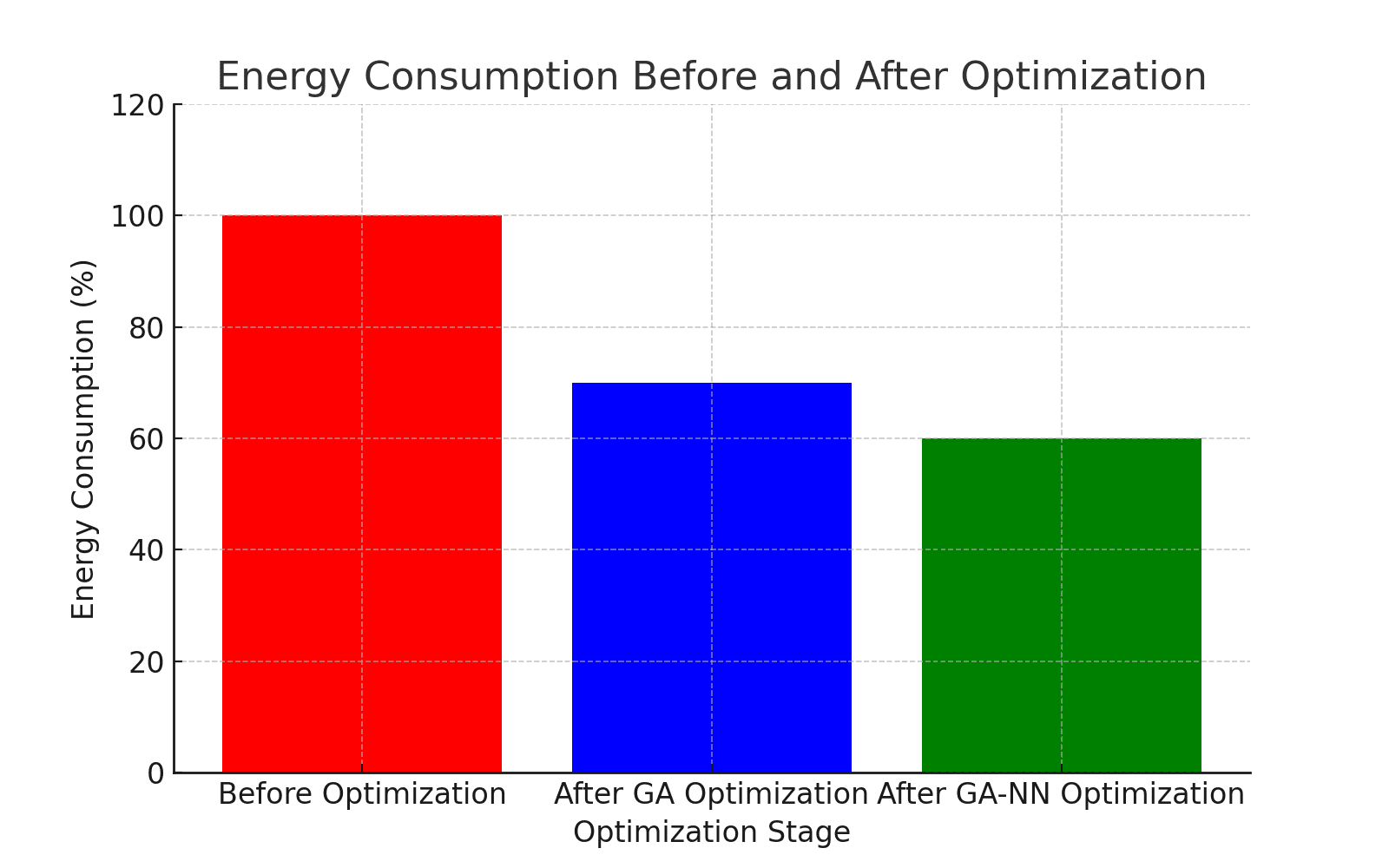
Chiller Optimization with Genetic Algorithms Basics
Genetic algorithms are techniques for optimization that emulate nature and the survival of the fittest. GA concerning absorption chillers uses the following parameters optimization:
- Evaporator temperature
- Absorber pressure
- Flow rates
- Heat recirculation exchanger efficiency
The Ways in Which Genetic Algorithms Operate
The algorithm is applied repeatedly until the correct answer is found. The steps are as follows:
1. Randomly select a solution from a certain area, this is known as population initiation.
2. Select the optimal result from the random result in the first stage, this is the solution optimization.
3. Create a new solution by merging the optimal selections of the survivors. This is known as crossover.
4. Mutation: Making small changes that broaden the search space.
5. Evaluation: Analyzing the new solutions to evaluate their effectiveness, and choosing the most successful solutions to carry forward in the next iteration.
By evolving possible solutions continuously, GA determines the optimal parameters that achieve maximum efficiency and energy conservation
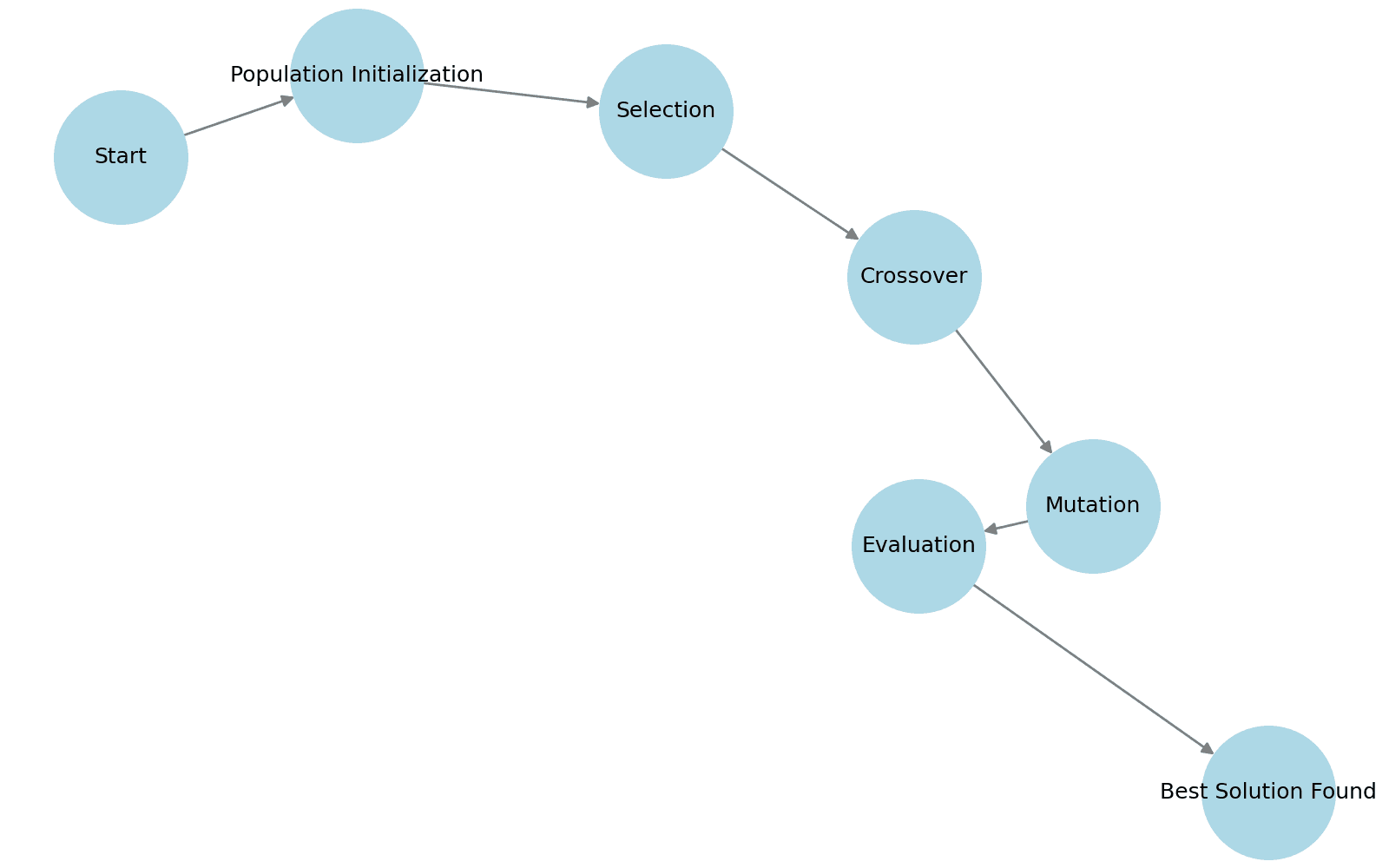
Case Study: Industrial Application of GA in Absorption Chillers
A 15% reduction in power consumption was achieved in a real-world scenario implemented in Japan’s energy sector. This was done by optimizing operational settings for the chiller using a GA based model. The system was able to modify setpoints dynamically to maintain optimal performance throughout different loading conditions.
Neural Networks: Intelligent Prediction and Control
The integration of Neural Networks (NN) with optimization techniques enhances the evolution of absorption chillers. NNs are capable of dynamically adjusting system parameters based on real-time monitoring of demand changes. Their key benefits include:
- Predictive anticipatory maintenance to eliminate breakdowns.
- Adaptive controls for ever-changing environments.
- Forecasted energy usage to further strengthen conservation efforts.
Example: AI Powered Chiller Optimization Peaked in Smart Cities
Absorption chillers installed in smart buildings across Singapore, powered by NNs, have seen a surge in efficiency by 20%. Such systems learn to automatically adjust for real-time climatic changes, driving down cooling expenses and improving user experience. The Hybrid Approach: Merging Genetic Algorithms With Neural Networks
The hybrid approach of integrating GA and NN presents a combined synergy. GA, through a simulation of natural selection, identifies the optimal initial parameters and ensures that only the best configurations are selected. After the parameters have been found, NN continuously updates those parameters through data and feedback, optimizing in real-time and adapting to new conditions. This partnership results in HVAC systems that self-optimize with little human control.
By employing GA, the proposed system can navigate through a wide range of inefficient solutions and prune the set of parameter configurations to the most effective. In turn, NN adds further optimized parameter configurations through learning patterns such as user behavior alongside thermic parameters like temperature and humidity. The longer the system runs, the more intelligent it becomes in predicting and adjusting the settings for the HVAC system.
Case Study: GA-NN Hybrid Model in a Manufacturing Facility
A leading manufacturing plant in Germany implemented a GA-NN hybrid optimization model for its cooling systems. The result:
• 30 percent reduction in operational costs
• Improved cooling for partially loaded systems
• Enhanced life span of equipment as a result of pre-emptive maintenance.
Future Trends in Absorption Chiller Optimization
1. AI-Powered Energy Management Systems: Integrating optimized chillers into smart grids.
2. IoT and Big Data Analytics: Enabling predictive control through monitoring performance in real-time.
3. Renewable Energy Integration: Employing waste heat from solar or geothermal energy.
4. Blockchain-Based Energy Trading: Cooling resources are decentralized and optimized freely.
5. Quantum Computing for Energy Optimization: Advanced simulations completed quickly and with ease.
6. Edge AIPowered Computing: Ultra-fast decentralized processing and real-time optimization.
7. Cooling solutions that use AI Optimization: The program minimizes the environmental effects of cooling technologies and energy use.
Practical Examples Of The Use Of AI For Optimizing Chillers
Example 1: Smart Commercial Building Cooling
An AI-based chiller optimization system was installed by a multinational corporation in New York, leading to a 25% annual saving in cooling costs. The system employed deep learning models to predict demand and optimize cooling cycles.
Example 2: Solar Powered AI Absorption Chillers Dubai
Dubai's sustainable energy programs featured solar-powered AI-optimized absorption chillers. This technology led to a significant reduction in carbon footprint as well as a 40% decrease in dependency on grid electricity.
Example 3: AI Chiller Maintenance in Hospitals
Implementation of an AI-based predictive maintenance system for chillers at a major London hospital was carried out recently. This implementation reduced equipment downtime to 50%, enhancing patient care.
Example 4: AI Cooling Chiller Networks in Data Centers
AI-powered chiller optimization was adopted in the Silicon Valley data centers. The modification resulted in a 35% decrease in energy consumption while ensuring optimal temperatures for the data servers.
Conclusion
The use of Genetic Algorithms and Neural Networks for the global optimization of absorption chillers represents a new modern cooling technology with greater energy-efficient capabilities. With the implementation of these technologies, industries stand to gain from reduced operational costs while becoming more sustainable, durable, and efficient in the long run. The future evolution of AI will further deepen its influence on HVAC system optimization, encouraging the development of advanced eco-friendly cooling technologies.
Frequently Asked Questions
What are absorption chillers, and why are they important?
Chilliers absorbs cooling and heating units compelling energy in the form of heat rather than electricity or gas. Furthermore, in the said process, electric energy is widely used in order to minimize off-shift waste energy, which makes them popular in industrial chiller HVAC applications.
Why do absorption chiller systems need optimization?
These processes use sophisticated thermodynamic cycles depending on the set system parameters and periodically changing workload conditions, environmental and energy consumption factors. Forcing optimization preserves energy costs, enhances efficiency, and therefore needs to lessen the negative impact on nature.
How can AI help optimize absorption chiller systems?
AI-based optimization techniques comprise chiller parameter adjustment based on defined and learned data collection of requirements and modification of operational settings predicted real time and needs efficiency deduction and maximization.
What is a Genetic Algorithm(GA), and how does it optimize chillers?
A Genetic Algorithm is a reasoning approach that mimics natural selection to improve performance. It uses multiple potential performance solutions to a single problem. The choices with the best results are used and are further optimized using crossovers and mutations.
In what ways do Neural Networks (NN) assist in the optimization of chillers?
Neural networks learn from real world data and adjust the system for optimal operations during the fresh air cooling season, while also forecasting future cooling needs and modifying system parameters for energy conservation.